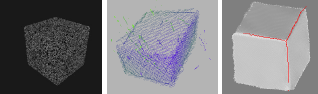
We present an integrated approach to the derivation of scene description from binocular and multiple-view stereo images, where both feature correspondence and surface reconstruction are addressed within the same framework. Special attention is given to the development of a methodology with general applicability. In order to handle the issues of noise, indistinct image features, surface discontinuities and half occluded regions, we adopt a tensor representation for the data and introduce a robust computational technique called tensor voting for information propagation. The key contributions of this paper are twofold. First, we introduce saliency instead of correlation scores as the criterion to determine the correctness of matches and the integration of feature matching and structure extraction; second, our tensor representation and voting as a tool enables us to perform the complex computations associated with the correct treatment of the stereo problem in three dimensions at a reasonable computational cost. We illustrate the steps on an example then provide results on both random dot stereograms and real stereo pairs, all processed with the same parameter set.